Summary
Object-level exclusions are a powerful feature in the Pure1® Data Protection Assessment tool. They enable you to create smart exclusion rules, which filter out unnecessary data and enhance the accuracy and effectiveness of assessments.
In classic video games, each item in your inventory serves a purpose on your quest. It’s the same in data protection, which requires an arsenal of tools to battle against threats. Just as choosing the right gear can make or break your adventure in a game, selecting which data to disregard and which to shield can be crucial in real-world applications.
The latest tool to know is object-level exclusions in Pure1, rules that can provide focus and clarity to your data resiliency efforts. Let’s explore how setting up these exclusions in Pure1 can allow you to customize your environment and focus only on the essential elements you need to succeed.
Why Object Exclusion Matters
In today’s data-driven world, ensuring the accuracy of your Data Protection Assessments is crucial. To achieve this, it’s essential to focus on the critical data that truly impacts your organization. An effective strategy is setting up object-level exclusion rules, which can significantly improve the accuracy of your assessments by excluding non-essential data such as “Test” and “QA” volumes, directories, filesystems, pods, buckets, or just any type of objects from skewing the results.
Tailoring Your Data Protection Strategy
As a Pure1 administrator, you have the ability to tailor assessments specifically to the needs of your organization. By setting up exclusion rules, you can filter out data stored in specific volumes, directories, filesystems, pods, buckets, or just any type of objects whose names contain certain keywords or phrases. This capability ensures that the assessment focuses solely on critical data, enhancing the reliability of your data protection strategy.
How Exclusion Rules Work
To customize your Data Protection Assessment, you can define rules based on specific criteria: Exclude data from assessment by specifying phrases or text patterns in the names of volumes, directories, filesystems, pods, buckets, or just any type of objects. For example, names starting with “Dev” or “QA” or containing “Testing” can be set to be excluded from the scoring process. On the Data Protection Assessments screen’s honeycomb view, you can click on the “Objects Excluded” hyperlink to see the pop-up to manage the exclusion rules.
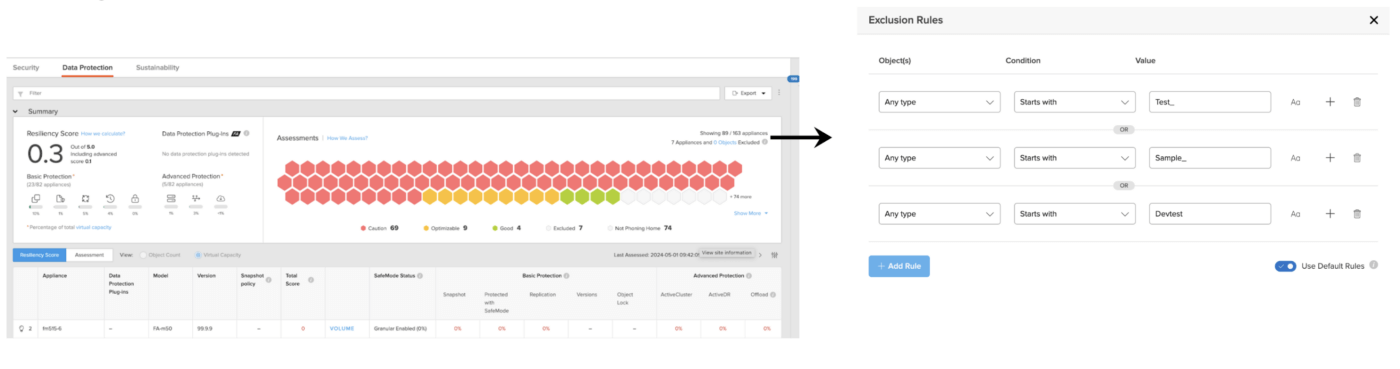
Figure 1: Pure1 Data Protection Assessment screen and Objects Excluded pop-up.
Admins can customize the exclusion lists based on organizational needs and review existing lists to ensure they are up-to-date. Changes to these lists can be made easily.
If the exclusion rules are modified, the system will display a confirmation dialog detailing the number of new objects to be excluded and the number of previously excluded objects to be reassessed under the revised exclusion rules. This provides admins with an opportunity to review the updated list of objects excluded before finalizing the changes by clicking “Save.”
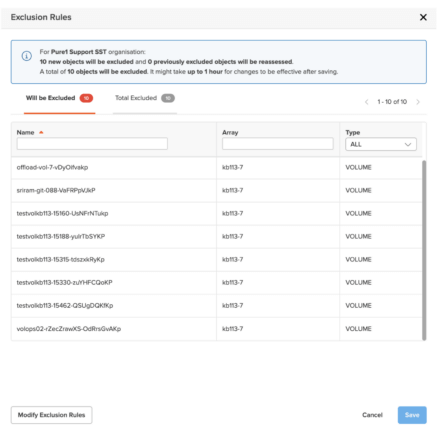
Figure 2: Setting up exclusion rules.
Presets
By default, certain exclusion criteria are already set up to help you get started quickly. The default criteria is anything that starts with “Test_,” “Sample_,” or “Devtest.” However, there is also flexibility to add more criteria to this default list or customize it as needed to better fit your organization’s data landscape. “By default, certain exclusion criteria are already set up to help you get started quickly. The default criteria is anything that starts with “Test_,” “Sample_,” or Devtest.” However, there is also flexibility to add more criteria to this default list or customize it as needed to better fit your organization’s data landscape.
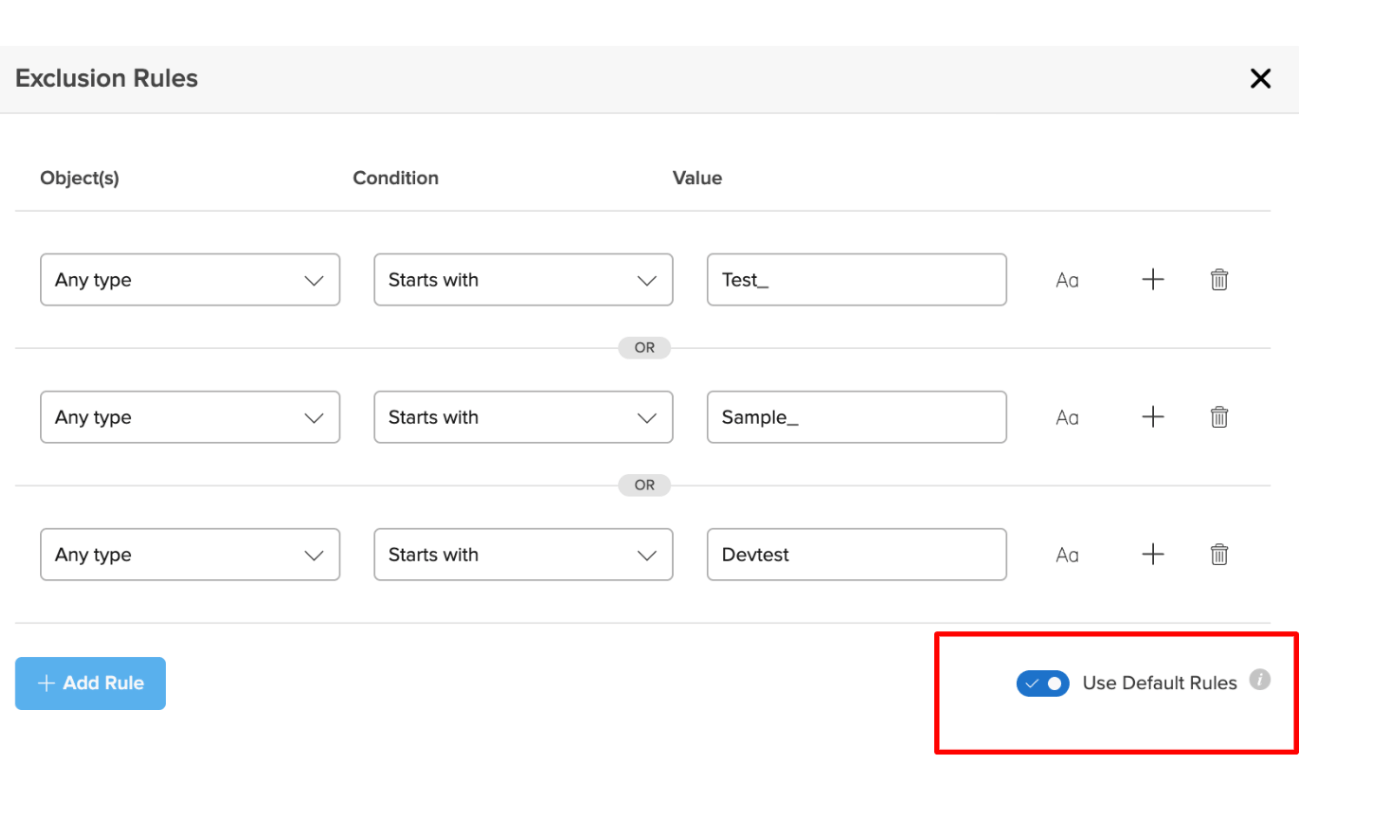
Figure 3: Selecting default rules for exclusion rules criteria.
By focusing on critical data and filtering out unnecessary data through smart exclusion rules, organizations can enhance the accuracy and effectiveness of their Data Protection Assessments. This not only improves data management but also ensures that data protection efforts are as efficient and focused as possible.

Written By: