Summary
Leveraging GenAI with RAG can deliver many benefits to financial institutions to fight financial fraud and enhance their compliance with KYC/AML regulations.
Know your customer (KYC)/anti-money laundering (AML) is a big and persistent challenge for financial institutions, and non-compliance can have huge impacts on a business. In October 2024, for example, one of North America’s largest banks was fined over $3 billion and saddled with ongoing business restrictions, including an asset cap, for failing to maintain a compliant AML program.
Fortunately, the emergence of generative AI (GenAI) coupled with retrieval-augmented generation (RAG) offers a powerful tool to improve and streamline these critical functions. Combining the capabilities of large language models (LLMs) with RAG, financial services companies can significantly enhance their KYC/AML workflows, leading to increased efficiency, accuracy, and scalability while reducing the firm’s risk. Here’s how.
Four Ways GenAI with RAG Revolutionizes KYC/AML
RAG augments standard LLMs with up-to-date proprietary and specialized data that has been cleaned and organized into vector databases to increase analytic accuracy while decreasing known issues with public LLMs like “hallucination.” As a result, key improvements include:
Efficiency: Streamlining Low-level Tasks
One of the most compelling benefits of GenAI is the ability to automate low-level, repetitive tasks in KYC/AML processes while simultaneously utilizing and shielding sensitive information by deploying RAG. Functions such as data collection, sanctions screening, and risk assessment are prime candidates for AI-driven automation. By leveraging LLMs, financial institutions can consume and analyze vast amounts of data at unprecedented speed and scale, far surpassing human capabilities. At the same time, utilizing RAG to incorporate curated data sets helps improve accuracy and depth of insights.
Pattern Recognition: Identifying Suspicious Behavior
GenAI excels at identifying patterns and anomalies in large and diverse data sets, making it an invaluable tool for KYC/AML. By training LLMs on historical data and incorporating RAG for contextual information, financial institutions can more effectively flag suspicious transactions, unusual behaviors, and high-risk customers. Moreover, GenAI can combine financial and non-financial events, such as location and time data, to provide a comprehensive view of potential risks.
Improved Accuracy: The Power of Quality Results
In addition to increased efficiency, a GenAI RAG solution can significantly improve the accuracy and quality of KYC/AML results. Traditional rules-based systems and human judgment alone often fall short in detecting complex patterns and hidden risks. By leveraging the power of RAG-enabled LLMs, financial institutions can achieve superior accuracy in risk assessment and decision-making. The human-in-the-loop interface, where AI-generated insights are reviewed and validated by compliance experts, ensures a superior blend of machine intelligence and human expertise. Over time, the AI models learn and adapt, continuously refining their performance and delivering more precise results.
Scalability: Handling Growing Volumes of Data
The exponential growth of data in the financial industry poses a significant challenge for KYC/AML programs. Traditional systems struggle to keep pace with the increasing volumes and varieties of information. Built on modern infrastructure, GenAI offers unparalleled scalability to handle these growing data demands. By automating data processing and analysis, financial firms can effectively manage larger data sets, including structured and unstructured data from multiple sources. This scalability enables comprehensive risk assessment and ensures that no critical information is overlooked.
Data, Models, and Efficiency Are Essential Areas of Focus
This approach delivers big gains in terms of efficiency, accuracy, and adaptability, but there are critical considerations that must be taken into account to maximize the potential of Gen AI with RAG while simultaneously mitigating risks and limitations. Broadly, there are three areas that require special attention:
Data
Security and quality are the two guideposts when it comes to data and GenAI with RAG.
For security, it is absolutely essential that sensitive, proprietary data such as personal information or proprietary analytical output be protected at all costs. Robust safeguards for data management, retrieval, and generation are essential.
With regard to quality, care should be taken when it comes to both data ingestion and the handling of conflicting or inconsistent information. In the first case, the best possible data is an absolute requirement as a raw input. In the second case, processes are needed to identify and mitigate instances when RAG data either conflicts with or is otherwise inconsistent with the data that was used to train the original GenAI model.
Models
As with data, care is required when it comes to the models that drive GenAI with RAG. Models should be customized to provide the best fit with the task at hand and consistently monitored and updated to maintain relevance and accuracy. The integration of RAG into an LLM may not always be straightforward either, necessitating work in the pre-training and fine-tuning stages of model development. Finally, the addition of RAG reduces, but doesn’t eliminate, challenges like hallucination or other inaccuracies, making model testing and monitoring all the more important.
Efficiency
There are three ways to look at efficiency when it comes to GenAI with RAG: data input, computational efficacy, and user interaction. With data input, there is a trade-off between RAG data and the LLM’s generative capabilities. Too much and there may be issues with overfitting or even an “echo chamber” effect, while too little may lead to suboptimal results. In terms of computational efficiency, it can be very costly to run non-optimized processes, especially with very large data sets. As Gartner highlights, it’s critical to choose the right type of storage deployment for your GenAI use case. Efficient indexing, retrieval algorithms, and caching help balance desired outputs against costs. Finally, user feedback and clarification requests are extremely valuable and should be considered when developing operational procedures.
Figure 1: Architectural overview of retrieval-augmented generation.
Pure Storage: Enhancing KYC/AML with GenAI and RAG
To harness the full potential of GenAI for KYC/AML activities, financial institutions need a reliable and high-performance data infrastructure. Pure Storage provides a validated, production-ready GenAI RAG solution for financial services that delivers performance and cost savings along with the necessary scalability, flexibility, and low-latency data retrieval to support the demands of KYC/AML programs. By leveraging Pure Storage, financial firms can ensure fast and efficient development of GenAI capabilities enhanced by RAG.
To address the critical objectives of KYC/AML regulations, which are to prevent money laundering and the financing of terrorism, financial institutions need to deploy the latest AI technologies. The benefits of using GenAI in KYC/AML processes are clear: Financial institutions can revolutionize their compliance efforts, reduce risks, and ultimately, better serve their customers. By leveraging the Pure Storage platform, enterprises can confidently embrace this innovative approach to KYC/AML, staying ahead of the curve in an increasingly complex regulatory landscape.
To learn more about GenAI with RAG for KYC/AML, download the latest white paper from Pure Storage
“Utilizing GenAI to Enhance KYC/AML and Fight Financial Fraud.”Fraud.”
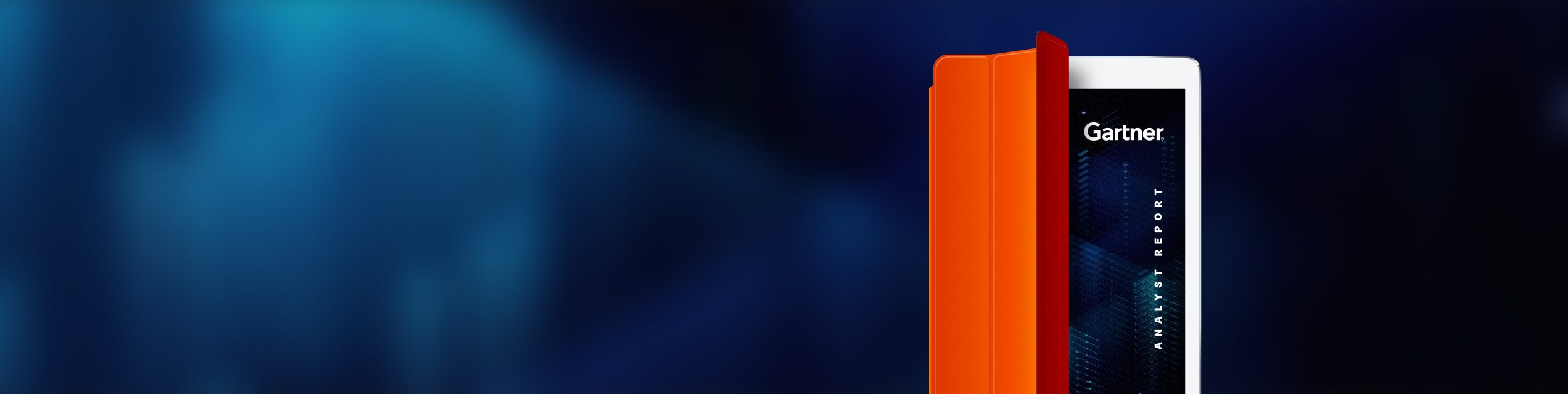
ANALYST REPORT,
Top Storage Recommendations
to Support Generative AI
Enhance Your Efforts
Learn more about using GenAI with RAG for KYC/AML to fight financial fraud.