Graphics processing units (GPUs) are the undisputed workhorses of AI projects. These small but dedicated cores are designed to perform thousands of calculations simultaneously. This massive parallelism makes them ideal for handling complex, data-intensive AI tasks, meeting the demands of AI and machine learning (ML) workloads at levels of speed and energy efficiency that central processing units (CPUs) simply can’t match.
Like most anything in high demand, GPUs are also in short supply. GPUs have been described as “rare Earth metals—even the gold—of artificial intelligence, because they’re foundational for today’s generative AI era.” (Fittingly, these powerful little units do contain gold, as well as other precious metals, like platinum.)
AI development is a top driver for the GPU shortage, especially with the rise of generative AI. But other factors, from geopolitical tensions to the popularity of PC gaming to cryptocurrency mining, have all been drains on supply. The race to acquire GPUs is poised to intensify as enterprises ramp up AI spending, which is projected to hit $500 billion by 2027. Some venture capitalists have even started stockpiling GPUs so they can rent them to AI startups in exchange for equity.
The takeaway: If your business needs GPUs to support its AI and ML projects, you’ll need to ensure you’re making the most of these precious resources at all times. With a future-proof and all-flash storage platform for AI and the right cost model, those GPUs won’t sit idle, and you won’t pay for idle data storage, either.
Securing More AI ROI
Even better, you can be confident about your return on investment (ROI), which is often elusive when investing in AI technology. The ROI certainty comes from instituting an AI-optimized infrastructure for data storage and processing that is sustainable and will continuously evolve as your AI initiatives grow—without disrupting productivity or innovation.
Here’s a closer look at why GPUs and an all-flash storage platform that serves as AI-ready infrastructure are a powerful team to help you achieve your AI and ML objectives.
- High-performance hardware like GPUs are only part of the complex tech stack that companies need to deploy AI projects. But they play a critical role in various layers of the stack, especially those requiring computational muscle. That includes the data processing and analytics layer, where GPUs can accelerate data preprocessing tasks like data cleaning and normalization, and the model training and optimization layer, where they can reduce the time needed to train models from weeks to mere hours.
- GPUs are only as fast or productive as the data they process. Data must be fed to data-hungry GPUs at blazingly fast speeds to prevent computational bottlenecks and inefficient data pipelines. All-flash storage, used strategically in multiple layers of the AI tech stack, can meet that need for speed. It can support the parallel, high-throughput data requirements essential for handling large-scale AI and ML workloads while optimizing performance, reducing latency, and providing reliability and durability.
A highly performant, best-in-class data storage platform like Pure Storage sets the stage for all of the above. Importantly, it unifies data into a single pool so it can be delivered to GPUs without delay, preventing them from idling while waiting for information.
Additionally, by freeing up data previously trapped on inefficient disk-based solutions on performant flash storage like Pure Storage, organizations can better address the ongoing challenge of meeting data volume needs for AI initiatives. This is no small thing. As this article about the race to amass data for AI underscores, the success of AI depends on data, and the more data that AI models have, the more accurate and humanlike they can become.
What’s Next for LLMs: Retrieval-augmented Generation
As for large language model (LLM) applications, which are trained on enormous sets of data and rely heavily on the massive computational power of GPUs for their training, retrieval-augmented generation (RAG) offers next-level optimization, availability, and speed. Pure Storage and NVIDIA provide a joint RAG solution that enables enterprises to improve and customize general LLMs with external, more specific, and proprietary data sources. NVIDIA GPUs are used for compute, while FlashBlade//S™, part of the Pure Storage platform for AI, provides all-flash enterprise storage.
Meet the First Storage as a Service for GPU Performance: Evergreen//One for AI
As your business scales its AI and ML projects and they become more sophisticated, you will need to rely on two crucial elements for success: more processing power and highly efficient storage capacity. That is especially true for AI initiatives that demand more energy for power-hungry GPUs, those precious resources that you want to maximize to the fullest.
Investing now in a robust and highly efficient storage platform that is optimized for AI will help ensure you’re ready to handle these demands in your data center—without the worry of hitting power constraints. Future-proofing your data center with Pure Storage’s validated architectures and Evergreen//One™ ensures not only high performance but also the ability to handle increasing AI workloads without the risk of hitting power constraints, thereby supporting continuous innovation and operational resilience.
Evergreen//One is the first storage-as-a-service (STaaS) solution purpose-built for AI. It provides guaranteed storage performance for GPUs to support training, inference, and high-performance computing (HPC) workloads. This SLA-driven storage service also simplifies the need for planning or overbuying by paying for throughput performance.
Think of this cost model like your monthly water bill. You pay a connection fee to guarantee a certain throughput of water to your home, and you pay a separate fee for water use. With Evergreen//One, the provisioned performance your GPUs require sets the benchmark for the throughput needed to support your AI training (i.e., your water connection pipe). Your usage is aligned to what you need for inference (i.e., how much “water” you actually use).
The results: You pay for what you need, and never overpay. And you ensure your GPUs—arguably the most expensive assets in your data center today—are always working hard for your money.
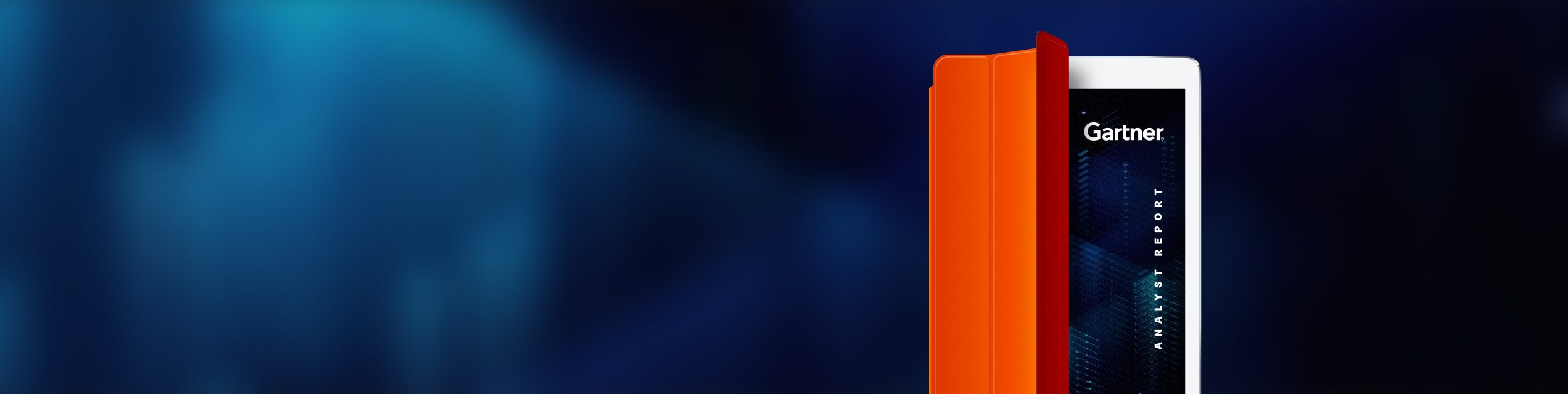
ANALYST REPORT,
Top Storage Recommendations
to Support Generative AI
A GPU Utilization Success Story: Chungbuk Technopark
One example of how data storage designed for AI can amplify GPU potential is the case of Chungbuk Technopark, a regional innovation hub that supports economic growth in the Chungcheongbuk-do province of South Korea. Chungbuk was facing resource constraints due to the GPU shortage, so it turned to Pure Storage for high-performance storage infrastructure.
With its new AI-optimized infrastructure from Pure Storage, Chungbuk achieved faster data access times for its AI workloads. This resulted in improved GPU utilization and accelerated model training times. Chungbuk was able to achieve its AI objectives. It also realized a twofold increase in storage data processing for faster AI performance.
See our ebook, Accelerate AI-driven Results, to learn more about how Pure Storage’s future-proof storage for AI is helping Chungbuk and other companies to overcome challenges in AI infrastructure deployments.
Read “Optimize GenAI Apps with Retrieval-augmented Generation from Pure Storage and NVIDIA” and Pure Storage’s OVX Certification announcement.
Power Your AI Compute
Learn more about the storage solution that pushes the boundaries of performance, scale, and efficiency.