This article on analytics innovation initially appeared on Kirk Borne’s LinkedIn. It has been republished with the author’s credit and consent.
We discussed in another article the key role of enterprise data infrastructure in enabling a culture of data democratization, data analytics at the speed of business questions, analytics innovation, and business value creation from those innovative data analytics solutions. Now, we drill down into some of the special characteristics of data and enterprise data infrastructure that ignite analytics innovation.
First, a little history—years ago, at the dawn of the big data age, there was frequent talk of the three Vs of big data (data’s three biggest challenges): volume, velocity, and variety. Though those discussions are now considered “ancient history” in the current AI-dominated era, the challenges have not vanished. In fact, they have grown in importance and impact.
While massive data volumes appear less frequently now in strategic discussions and are being tamed with excellent data infrastructure solutions from Pure Storage, the data velocity and data variety challenges remain in their own unique “sweet spot” of business data strategy conversations. We addressed the data velocity challenges and solutions in our previous article: “Solving the Data Daze: Analytics at the Speed of Business Questions.” We will now take a look at the data variety challenge, and then we will return to modern enterprise data infrastructure solutions for handling all big data challenges.
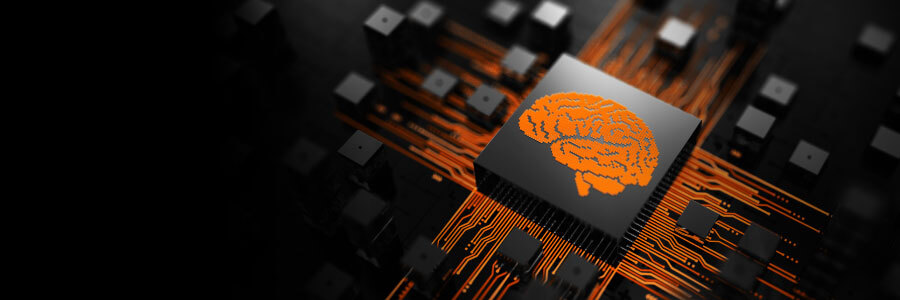
Customer Journeys to AI Success
Data Variety Is a Big Analytics Challenge
Okay, data variety—what is there about data variety that makes it such a big analytics challenge? This challenge often manifests itself when business executives ask a question like this: “What value and advantages will all that diversity in data sources, venues, platforms, modalities, and dimensions actually deliver for us in order to outweigh the immense challenges that high data variety brings to our enterprise data team?”
Because nearly all organizations collect many types of data from many different sources for many business use cases, applications, apps, and development activities, consequently nearly every organization is facing this dilemma.
Orchestrating analytics and insights discovery across diverse, distributed data sources is hard enough, but especially so if that data is hard to find, hard to access, and burdened with its own data delivery latency bottleneck from the source to the end user. Distributed data sources can create friction for data teams when attempting to integrate multiple data sets. That’s a big problem for analytics innovation since those high-variety data sets promise—when combined—to yield deep, actionable insights that create new value for organizations. One way that I have described this particularly positive characteristic of data variety is this: Variety is the spice of discovery! Here are three common examples:
- Data variety enables the use of data features from multiple data sources to disambiguate two different entities (e.g., customers, products, events, behaviors, cyber actors) that would otherwise appear to be the same when viewed in a small number of “low information” features within a single data source. Data variety can thereby significantly improve analytics model accuracy—reducing false positives, false negatives, and other misclassifications.
- Data variety enables the use of multiple data features to detect when two different entries in different data sources are actually referring to one and the same entity (e.g., the same customer in the marketing database, sales database, customer call center CRM database, and product returns database).
- Data variety enables the discovery of new classes and categories of entities and events—exploring the high-dimensional data space to uncover new types of entities and events in your domain that were previously not identified as such because the data space was unintentionally being projected into a lower dimensional view, using too few data features, thereby yielding a biased projection of a more complex and diverse data space of the sample population.
High-variety data lives in a high-dimensional data feature space that also includes real space (geospatial, location-based data features) and real time (e.g., time series, streaming sensor data, time-of-day labels on data, etc.). An example of a business analytics application where these features are especially important is marketing when making personalized location-based time-dependent product recommendations to a customer.
To summarize what we have just described regarding the “what” and “why” of big data variety, we will use a rocket science metaphor: The data infrastructure of an enterprise may not be a “starship,” nevertheless it really does represent a data space-time continuum (a federation of high-variety data features) that can ignite and accelerate analytics innovation for stellar business growth.
Modern Enterprise Data Infrastructure Solutions Can Handle Big Data Challenges
Now, what about the “how” of big data variety—how can an enterprise deal with its challenges?
We find that Pure Storage provides uniquely powerful solutions for the high-variety big data challenge. First and foremost, from a strategic perspective, Pure Storage makes this possible because it’s a foundational data platform that can support many types and modalities of data (structured and unstructured), recognizing that data variety is critical to the analytics strategy, applications, and infrastructure of the modern organization. Next, from a tactical (practical applications) perspective, we recognize that Pure Storage products can handle data variety in impressive ways.
For example, typically an organization would need different types of data storage devices for different kinds of data types. That can then lead to isolated data silos—a frequent cause of unsuccessful data strategies and broken analytics applications. Pure’s storage platform can handle the variety of today’s data: structured, semi-structured, unstructured, file, block, object, streaming/batched, small files/really large files, etc.
Pure Storage solutions can also parallelize the data operations. This capability is a game-changer in simplifying data and analytics operations as well as speeding time to insights. Parallelism is also an essential benefit of the data infrastructure when there are many users, use cases, and applications running data in and out of the storage system. With Pure Storage, fragile data staging orchestrations (discovery, access, delivery, integration) across distributed sources are no longer required for complex multi-data set correlations. Pure Storage solutions greatly simplify data staging and make it much more robust, reproducible, and transparent, so that data scientists can spend more time in the knowledge and insight layer, and less time in the IT layer.
That’s “how” enterprise leaders and data practitioners learn to love data variety.
Read More from This Series
Pure Storage data infrastructure solutions keep high-variety data analytics processes running smoothly and continuously, especially when low-latency discovery and response are critical. On-prem business analytics applications and solutions require data to be transported across the data space-time continuum at “starship enterprise” speed. That requires on-prem data infrastructure solutions that are analytics-ready and AI-ready. Learn more about how this is already happening for Pure Storage customers in the following case studies within these different domains:
- Equity trading markets
- Infrastructure as a service (IaaS)
- Commerce and customer service
- Financial
- Medical research
Read our two related articles in this three-part series focused on enterprise analytics innovation:
- Solving the Data Daze: Analytics at the Speed of Business Questions
- Delivering Low-latency Analytics Products for Business Success

Written By:
Data at Warp Speed
Learn how Pure Storage analytics and AI solutions can help accelerate time to insights.