Summary
While AI is powering exciting breakthroughs, ensuring AI systems are ethical, reliable, and compliant poses a challenge. AI data governance is a framework of policies, processes, and practices designed to ensure that the data used for AI models is accurate, secure, ethical, and compliant with regulatory requirements.
Ever heard the phrase “garbage in, garbage out”? That’s exactly how AI works in terms of the data used to train it. If bad (ie, inaccurate or incomplete) data goes in, bad AI will come out. If good (ie, accurate and complete) data goes in, good AI will come out. By “good” AI we mean fair and precise.
The problem is the complexity and amount of data used to train AI and machine learning models. It’s a lot to manage. Hence the need for AI data governance, which is basically just the ways – meaning policies and processes – organizations ensure the data they feed into their AI models is secure, accurate, relevant, and thorough.
Good AI data governance improves model performance, increases reliability, builds trust, and leads to ethical AI outcomes that aren’t biased in any way. All major wins, right?
Read on to explore all the essential elements of AI data governance for training data, including:
- The key principles and objectives of AI data governance for training data
- Common challenges in AI data governance
- Best practices for establishing effective AI data governance frameworks
Key Components of AI Data Governance
It’s probably not hard to imagine what comprises data governance, but defining and exploring these terms could be helpful.
There’s general “data quality”, of course. This could be very subjective, but it essentially comes down to data consistency, completeness, and correctness, meaning the elimination of errors, duplicates, and irrelevant information. Quality also involves inappropriate data. As an example, this was a while back but in 2016 Microsoft’s AI chatbot Tay turned into a PR disaster when it began to spit out racist responses. Why? Because it had learned its values and language from Twitter.
Good data governance also prioritizes compliance with privacy regulations like GDPR or CCPA. Auditing is here to ensure data anonymization and minimization, user consent, and transparency about data usage. GDPR fines may have dipped, but that doesn’t mean organizations shouldn’t still be vigilant.
Security is another major aspect of AI data governance. Protecting sensitive and proprietary training data from unauthorized access or breaches involves implementing robust encryption and access control mechanisms and monitoring for vulnerabilities and unauthorized data usage. Companies also need to ensure secure storage and transmission of training data sets.
AI Data Governance Challenges
Certain common things tend to make AI data governance challenging.
We’ve all heard of “data silos”, for example. Data silos make it harder to cohesively manage AI training data, leading to inconsistencies and inefficiencies. What can help with data silos? Implementing centralized data repositories or data lake architectures to consolidate data sets. You can also use data integration tools and platforms to streamline access and ensure consistency.
Lack of standardization is another issue. Diverse data sources and formats can make it difficult to manage and govern effectively reducing interoperability and complicating preprocessing and training workflows. Sometimes it’s hard just to know where your data is coming from. Standardizing formats, labeling, and metadata can go a long way to making this easier. You can also develop robust documentation practices and maintain a clear audit trail for all data processes. Use tools that track data lineage, transformations, and usage across the AI lifecycle.
Also the sheer amount and variety of training data required for AI and ML models can be staggering. Unstructured data, for example, (e.g., text, images, video) presents its own set of issues for storage and analysis. Investing in scalable infrastructure such as cloud-based platforms and tiered data storage can help with this.
Conclusion
Remember: garbage in, garbage out. Ensuring you aren’t feeding your AI models garbage is a company-wide effort requiring massive cross-collaboration. This kind of goes without saying but your company should have comprehensive policies covering data collection, storage, usage, and retention.
A huge part of all of the above is having the right data infrastructure to support your AI initiatives. The Pure Storage platform helps organizations maximize performance and efficiency, unify their data, simplify data storage management, and solve the unpredictability of AI growth. Pure Storage® FlashBlade® is a certified storage solution for NVIDIA DGX SuperPOD, and Pure Storage was among the first enterprise storage vendors to work with NVIDIA on certified AI-ready infrastructure solutions that expand and accelerate the adoption of AI.
Learn more about how you can future-proof and accelerate your AI results with Pure Storage.
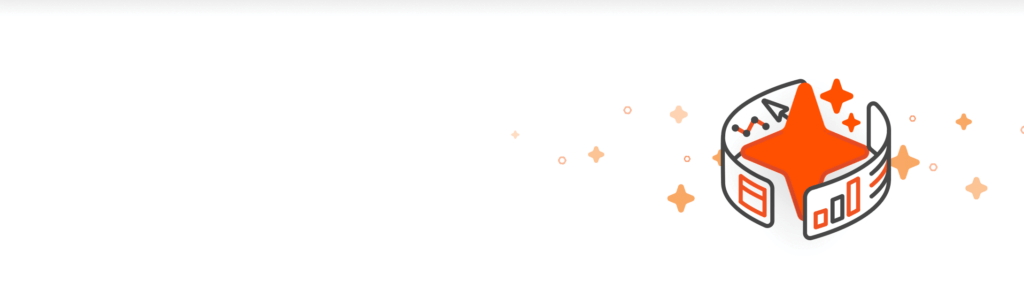
Pure Storage Business White Paper
The Pure Storage
Platform for AI accelerates
and simplifies AI deployments
Power AI Success
Learn how Pure Storage can help you accelerate your AI results.
