Can explainable AI help us overcome the ‘Black Box Problem’ and build more trustworthy AI? Consider this: When you use an AI-powered recommendation engine to view options on streaming entertainment services, you likely don’t care how those recommendations were generated. But when AI helps decide if your business gets a loan, who you hire, or which customers you accept or reject, you and other business leaders need to know more about how decisions get made behind the scenes. Depending on the organization or market, customers may also demand to know how your algorithms make their magic or where your data comes from.
As it turns out, AI developers and researchers have been taking a hard look at perceptions of trustworthiness of AI. For AI to fulfill its potential, it must be trusted both in the abstract (as an ethical and market imperative) and by everyday users who need AI to make critical decisions in real time. Explainable AI (XAI), a way of designing and building AI systems, aims to provide transparency, interpretability, and explainability along with the desired results.
Explainable AI has four characteristics, according to the National Institute of Standards and Technology:
- Explanation: A system delivers or contains accompanying evidence or reasons for outputs and/or processes.
- Meaningful: A system provides explanations that are understandable to the intended consumers.
- Explanation accuracy: An explanation correctly reflects the reason for generating the output and/or accurately reflects the system’s process.
- Knowledge limits: A system only operates under conditions for which it was designed and when it reaches sufficient confidence in its output.
The Benefits of Explainable AI
The benefits of explainable AI may go beyond improving the AI user experience—providing essential feedback for further model training and enabling human learning beyond the decision at hand. In a sense, XAI may hold the key to future harmony between technology and its users.
Until now, establishing trust in technology was primarily about simply winning users over by convincing them of the usefulness of the solution. This buy-in is easily achieved in part because we usually don’t need to understand in detail how a given technology works to see how it helps us. In other words, it’s not a “black box”—the term for an opaque technological process that denies explainability.
But with the rise of AI, we’re being asked to trust such black boxes more than ever.
Shouldn’t All AI Be Explainable AI?
If XAI sounds like a useful approach, why not build all AI as XAI?
To begin with, for much of the basic AI we already use, explainability simply isn’t needed or desired by users—for example, the AI used for recommendation engines or voice recognition. There are also applications where it wouldn’t be practical to enable XAI, such as the results of massive computations and/or algorithms with large numbers of variables, as with autonomous vehicles.
In many cases, XAI may be more useful as a development tool than as a part of production environments. XAI can help speed up the model training process by providing feedback that can be used to debug, fine-tune, and optimize an application.
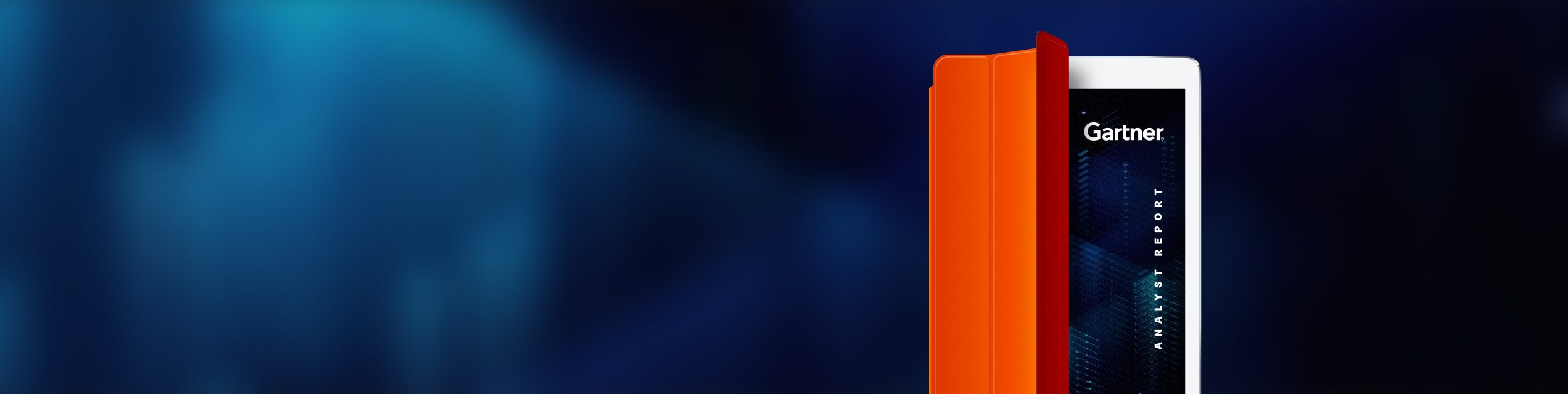
ANALYST REPORT,
Top Storage Recommendations
to Support Generative AI
XAI Benefits Don’t Come Cheaply
XAI can be difficult to engineer, requiring significant features and resources that could reduce the performance of the application. XAI can also become a drag on compute resources, a significant factor in today’s environment, where such resources are increasingly stretched and increasingly valuable. A disproportionate focus on XAI runs the risk of impeding overall development of AI.
AI developers are concerned about other downsides to transparency. For example, XAI could provide the means for scammers and cybercriminals to game systems for their own benefit. Another concern is that, by revealing too much about its algorithms, XAI might expose its intellectual property to competitors who could replicate the data sets and algorithms.
When XAI Is Better AI
There are some use cases where XAI will likely become a default feature. One of the best examples is medical diagnostics, where medical imaging is analyzed by AI to direct treatment. For this methodology to gain acceptance, it must be trusted by doctors who are making life and death decisions.
To establish this trust, doctors need to understand the insights derived from AI. One solution is for the AI to enhance the diagnostic imaging, highlighting the areas and visual data that influenced the decision. This solution not only helps explain how AI came to its diagnosis, but it’s also a powerful tool for enriching doctors’ knowledge.
In the future, privacy regulations may drive the spread of XAI. The EU General Data Protection Regulation (GDPR) already guarantees consumers “a right to explanation.” As more and more decisions are made by AI, consumers will seek explanations and transparency.
Adopt the Last AI Storage Platform You’ll Ever Need
Add GPUs to your compute farm with confidence, knowing that you can tune and upgrade your storage non-disruptively. Pure’s unique Evergreen® technology and services ensure that our products never become obsolete, never need to be replaced, and can go through non-disruptive upgrades. Through our Evergreen subscription portfolio, you upgrade the hardware and software in your AI storage systems non-disruptively, forever.

Written By:
Stay Agile
Upgrade the hardware and software in your AI storage systems non-disruptively, forever.