Summary
AI workloads require substantial processing power, which requires high electricity usage. As AI becomes more widely used, reducing AI power consumption can help businesses save money and improve sustainability.
Are we on the brink of an AI energy crisis? That seems to be the direction things are heading. Data centers consume vast amounts of electricity, and as AI adoption takes off, the problems associated with high power consumption will undoubtedly grow much worse. After all, AI is computationally intensive, and its application in business is increasing exponentially.
A recent survey conducted by Pure Storage in partnership with Wakefield Research found that nearly half of the companies that have adopted AI have doubled their computing power or more since adopting AI. Of those, 73% found themselves not fully prepared for the associated increase in energy requirements.
Reducing power consumption mitigates the negative impact of data centers on our environment, and it keeps operational costs from spiraling upward. Efficient power usage enables businesses to scale effectively, expanding AI adoption without running into insurmountable obstacles such as capacity limits or prohibitive energy costs. High power consumption also generates heat, so a reduction in energy usage also lowers the load on cooling systems.
As companies look to save money and improve their environmental sustainability scores, a reduction in AI power consumption presents a clear win-win opportunity.
Understanding AI Power Consumption
The computational intensity of AI workloads, particularly for training ML models and deep learning models, requires substantial processing power, which in turn leads to high electricity usage. These models often involve complex algorithms and very large data sets, requiring specialized high-performance hardware.
The constant need for data retrieval, processing, and storage in real-time AI applications adds even further to energy consumption. The infrastructure supporting these operations, including cooling systems and backup power supplies, further increases the load.
To evaluate overall energy efficiency, data center managers use a widely recognized metric known as power usage effectiveness (PUE). This is the ratio of total energy consumption at the facility to the energy consumed by IT equipment alone. A lower PUE indicates greater efficiency, meaning a larger proportion of energy is used directly for computing rather than supporting infrastructure. In the context of AI operations, optimizing PUE is essential because the high energy demands of AI workloads can exacerbate inefficiencies.
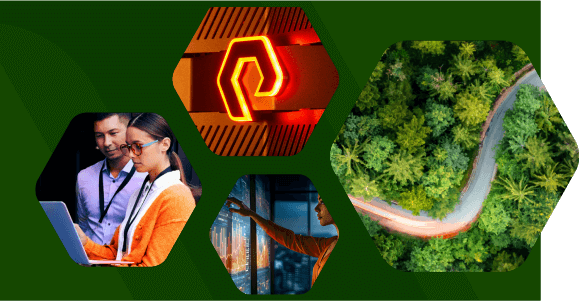
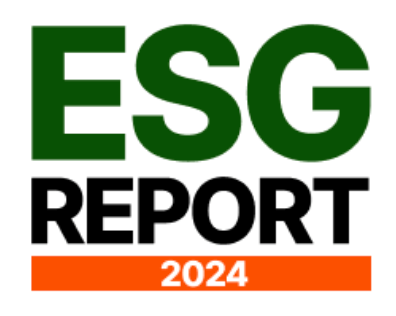
Optimizing AI Hardware Infrastructure
Selecting energy-efficient hardware components is a critical first step for AI data centers aiming to reduce power consumption and improve sustainability. Look for servers and processors with high-efficiency ratings and features such as power scaling, which adjusts power usage based on workload demands. Consider hardware that supports virtualization, which allows more processes to run on fewer physical machines, thereby saving energy. Investing in advanced cooling technologies, such as liquid cooling, can also enhance overall energy efficiency by reducing the need for power-intensive air conditioning systems.
Specialized AI processors and GPUs also offer significant benefits because they are engineered to handle highly demanding AI workloads more efficiently than general-purpose processors. Parallel processing enables them to perform AI calculations faster and with less energy consumption. For example, when tasks are distributed across multiple processors, each processor can run at a lower clock speed. Power consumption is proportional to the square of the clock speed (P ∝ f²). Therefore, reducing the clock speed results in a significant reduction in power consumption. This not only reduces power usage but also accelerates AI training and inference processes, leading to faster and more cost-effective operations. By leveraging these specialized components, data centers can achieve higher performance while maintaining lower energy consumption.
Modern all-flash storage solutions for AI provide considerable advantages for both performance and power efficiency. All-flash storage systems use solid-state drives, which consume significantly less power compared to traditional disk drives, and offer much faster data access speeds. The improved efficiency of all-flash storage means that less energy is required to achieve the same or better performance levels, leading to cost savings and a smaller environmental footprint.
Efficient Cooling Solutions
Cooling represents a significant portion of a data center’s energy usage, often accounting for nearly half of overall power consumption. There are several strategies that data centers use to achieve efficient thermal regulation.
Hot aisle/cold aisle containment involves organizing server racks into rows with alternating hot and cold aisles. Cold air is directed to the front of the servers through the cold aisles, while hot air is expelled through the hot aisles and contained, preventing it from mixing with the cold air. This configuration enhances cooling efficiency by maintaining a consistent airflow and temperature, reducing the workload on cooling systems.
Liquid cooling uses water or other coolants to absorb heat directly from IT hardware. This is significantly more efficient at transferring heat and can handle higher thermal loads with less energy. Liquid cooling systems can be integrated directly into server racks or deployed as part of the overall cooling infrastructure, providing targeted and efficient heat removal.
By implementing these advanced cooling strategies, data centers can achieve substantial energy savings and reduce their reliance on power-hungry air conditioning units.
Power Management Techniques
Power management techniques like dynamic voltage and frequency scaling (DVFS) can also improve the energy efficiency of data centers. DVFS enables processors to adjust their voltage and frequency according to workload demands. This requires hardware that supports DVFS, and administrators must configure operating systems to enable it. By using DVFS intelligently, you can reduce power consumption during low-demand periods without sacrificing performance during periods of peak activity.
Workload consolidation and resource allocation optimization can also be effective in reducing power consumption. Workload consolidation involves running multiple workloads on fewer servers, maximizing server utilization, and allowing idle servers to enter low-power states. Resource allocation optimization ensures computing resources are allocated efficiently, preventing waste.
Data Center Design Considerations
The design of a data center plays an important role in reducing AI power consumption. By optimizing airflow, cooling, and electrical distribution, data center operators can maximize energy efficiency.
We’ve already described strategies such as hot aisle/cold aisle containment, but there are other design strategies that can help you achieve AI data center energy reduction. For example, modular data centers built using prefabricated units allow for incremental expansion and reconfiguration, enabling data centers to scale their operations without over-provisioning resources. Modular designs also facilitate better cooling and power distribution, as each module can be optimized for specific energy-efficient configurations.
Renewable energy sources can also reduce power requirements and environmental impact. Solar, wind, and other renewables can support corporate sustainability goals while providing a reliable and often cost-effective energy supply for AI data centers.
Monitoring and Analytics
Real-time monitoring and analytics help data center managers identify power consumption patterns, pinpoint inefficiencies, and understand peak usage times. This allows for more informed decisions on optimizing energy use, such as adjusting cooling systems, consolidating workloads, and implementing power-saving features. Real-time data helps in proactively managing power consumption, ensuring that resources are used efficiently and that costs are kept under control.
In today’s smart data centers, AI is offering solutions to the problem, helping forecast future power demands and identify potential issues before they become critical.
Pure1® AIOps, for example, uses predictive analytics to help data center managers better understand performance and capacity needs, optimize energy efficiency, and secure their critical data. Pure1 AIOps provides comprehensive monitoring and predictive maintenance for storage arrays, leveraging AI to analyze performance data and predict potential issues.
Today’s organizations need to stay ahead of the curve with an agile, AI-ready infrastructure that supports AI orchestration. Pure Storage provides products and solutions that help today’s most innovative organizations keep up with the large data demands of AI workloads. Pure Storage facilitates faster, more efficient, and more reliable model training and contributes to optimizing the machine learning pipeline. That leads to better performance and productivity for data-driven initiatives of all kinds.
Want to learn more? Contact the experts at Pure Storage today.

Written By:
Contact Us
Learn more about how Pure Storage can help you build a greener data center.