Summary
Data storage is an essential layer in the GenAI stack, but not all data storage solutions have the capabilities necessary to support it. Pure Storage solutions offer everything you need for headache-free data storage for AI workloads.
Summary
Data storage is an essential layer in the GenAI stack, but not all data storage solutions have the capabilities necessary to support it. Pure Storage solutions offer everything you need for headache-free data storage for AI workloads.
Generative AI, including large language models (LLMs), is a whole new beast for organizations—especially for IT—requiring new ways of storing and managing data. Most enterprises are just beginning to assess their data storage needs for generative AI.
There are certain questions you’ll want to ask about your data storage needs as you prepare for the AI onslaught, including: Is my current data storage solution and infrastructure cost-optimized and ready for whatever the future may bring?
Infrastructure and operations (I&O) leaders are primarily evaluating their AI and GenAI infrastructures from a memory and compute performance perspective. However, they can frequently overlook data storage in this process, even though it’s an essential layer in the GenAI stack that can become a bottleneck during model training. Storage with insufficient consistent throughput can slow down the data feed to GPUs and hinder model checkpoint and recovery processes, wasting valuable compute resources.
One telling fact per Gartner: By 2028, three-quarters of organizations with generative AI training data will deploy single storage platforms to store it, up from 10% in 2024.
What’s behind this trend? Let’s look at the unique data storage requirements for generative AI to learn more.
Read the Analyst Report: “Top Storage Recommendations to Support Generative AI” >>
What Type of Data Storage Can Support Generative AI?
“One size fits all” may work for some things, but it won’t work when selecting an effective storage infrastructure for generative AI workloads. The workflow requirements are simply too diverse. That and large-scale generative AI deployments will require distinct storage performance and data management capabilities. Most legacy storage is not performant enough.
The good news? Most enterprises won’t have to build an entirely new AI infrastructure from scratch to leverage generative AI because they likely will be fine-tuning existing open source LLMs instead of training new ones.
Source: Gartner
That said, I&O leaders will need to focus on having not only high-performance storage for training those models but also a comprehensive end-to-end workflow strategy, including RAG pipelines.
Most organizations will either adopt an existing AI model or retrain one using their business data, possibly supplemented with external data tailored to their needs. However, they’ll still need to rethink their data storage strategy.
The analyst report from Gartner shares specific storage recommendations for organizations leveraging generative AI, including three unique capabilities:
- A scalable data lake storage platform that can handle all data used for model training. A storage platform should be able to accommodate various use cases including file- or object-based, throughput- or latency-sensitive workloads, large or small files, and metadata-heavy or data-access-heavy workloads.
- Features that ensure high performance to keep GPUs engaged during training and complete model checkpoint and recovery processes efficiently. Insufficient data feed to GPUs results in idle GPUs, equating to wasted costs.
- Global data management capabilities across on-premises deployments, multiple clouds, and edge locations. Without these capabilities, data required for training or refining a model must be copied, leading to operational complexity and wasted capacity.
Modernizing storage is essential to achieve these capabilities and is particularly urgent for enterprises training new LLMs on large-scale data. As AI data demands grow, Evergreen//One™, a storage-as-a-service offering, offers scalable architecture that can handle increasing workloads efficiently, providing consistently high performance crucial for fast data processing and low latency. As sustainability becomes even more of a priority for organizations, Evergreen//One eliminates wasteful overprovisioning and includes the industry’s first energy efficiency SLA.
For enterprises testing existing LLMs with small data sets, an all-in-one, full-stack, GenAI-in-a-box converged storage solution such as FlashStack® for AI or AIRI//S™ may be ideal. These solutions provide the necessary compute, storage, and networking infrastructure along with an off-the-shelf pretrained LLM.
Conversely, organizations with unknown compute or storage needs, and no restrictions on storing data in a public cloud, may find a full public cloud solution more suitable.
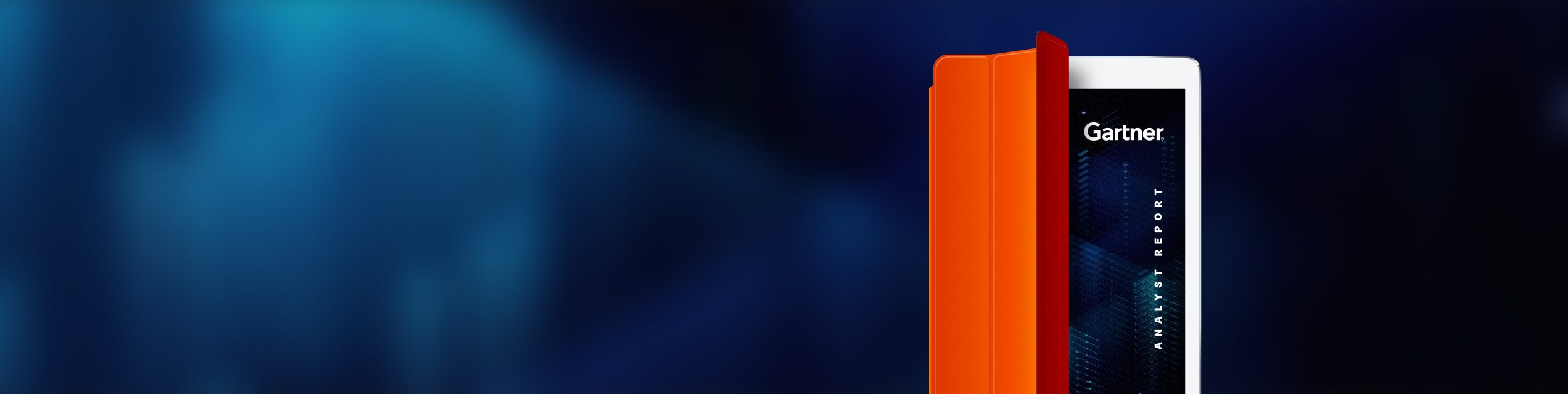
ANALYST REPORT,
Top Storage Recommendations
to Support Generative AI
The Pure Storage Platform for Generative AI Workloads
Per the above, a few things are clear about data storage needs for generative AI workloads. Data storage needs to be:
- Highly performant to support different types of workloads (i.e., sequential, random, batch, etc.)
- Consolidated to a single platform to eliminate bottlenecks and deliver high performance
Pure Storage offers both and puts enterprises in the best possible position to future-proof their AI storage. Evergreen//One can significantly future-proof AI investments by ensuring continuous, non-disruptive upgrades, which maintain state-of-the-art infrastructure without downtime. Evergreen//One provides predictable costs, eliminating large capital expenditures and allowing better budget allocation, which is key when making investments in AI.
Download the report to learn more, and explore how the Pure Storage platform helps you accelerate AI adoption.
Written By:
Dive Deeper
Learn more about the top storage recommendations to support GenAI.