Diagnostic analytics is also known as root cause analysis. While it’s a data-driven field that’s useful in every industry, there’s arguably no more important application for diagnostic analytics than applying AI in healthcare. The goal of healthcare analytics solutions is to discover the root cause of why something happened the way it did. This begins with data analysis but often requires a much more in-depth examination, which is where AI in healthcare shines.
AI has the ability to process and analyze massive amounts of data. AI programs can speedily digest thousands of scholarly medical research articles and reports, make connections, and identify patterns that no human ever could. Some of the most common techniques include employing algorithms, data discovery, data mining, filtering, probability theory, and sensitivity and statistical analysis. These tools are used to detect anomalies, isolate patterns, and determine causal relationships. Because of the massive amount of information used in multiple levels of investigation, a machine learning system that can work through terabytes of data is very beneficial.
The Purpose of Diagnostic Analytics in Healthcare
Healthcare organizations use diagnostic analytics platforms to understand why things happened—for instance, why a patient had to be hospitalized or why a particular treatment didn’t work as expected. Diagnostic analytics can be used to gain insights into any and every aspect of healthcare, from cost and billing to the efficacy of surgical techniques and reinfection rates with certain kinds of treatment. AI-based healthcare analytics solutions have gained traction at many leading hospitals worldwide. In the United States alone, AI healthcare applications are projected to save the medical economy an astounding $150 billion annually by 2026. Much of this will be done by leveraging the impressive power of AI.
One of the more common AI applications of healthcare diagnostics is chatbots. Although consumers can experience low-powered versions of these on all kinds of websites, the type used to power effective healthcare analytics solutions is much more sophisticated. These chatbots are trained on enormous healthcare data sets to recognize disease signs and symptoms, markers, and available treatments. By interacting with patients, the chatbot can serve as an effective first point of contact and refer patients to virtual connections with a nurse, for instance, or identify patients who should immediately see their doctor in person.
Physicians are incredibly busy, and keeping up with the latest research, treatment techniques, and medical innovations is often a priority that falls by the wayside. Using AI-based diagnostics platforms, physicians can access the most up-to-date medical knowledge in a user-friendly format that can be easily presented to patients. This can help improve the accuracy of diagnosis in general, as well as the patient experience and outcomes.
What Are Examples of Diagnostic Analytics in Healthcare?
Diagnostic analytics in healthcare can help organizations in many ways. Some examples include determining:
- The most common signs and symptoms of a specific illness
- Why some patients don’t follow through with home therapy and others do
- Which patients are more likely to follow prescribed treatment plans
- Why one doctor’s surgeries always go over the estimated time
- Who is most vulnerable to complications after surgery
- Which populations are more likely to contract certain illnesses
- Who is using their prescriptions and who isn’t
However, not all applications of diagnostic analytics relate directly to patient care. Just as important are the supporting details that enable effective treatment, and healthcare analytics solutions provide tremendous value here. They can help healthcare organizations detect fraud risk, streamline the insurance claim journey, forecast patient loads, determine optimal pricing models, compete more effectively with other organizations, and much more.
What Is the Future of Healthcare Diagnostic Analytics?
AI in healthcare can be employed in virtually every arena where data is present. Diagnostic analytics solutions will continue to improve as AI and machine learning technology evolves. These solutions will facilitate increasingly more effective patient care by providing organizations with the tools to get rapid and accurate insights from data—leading to improved outcomes, speedier recoveries, and more satisfied patients while avoiding potential pitfalls. These diagnostic analytics tools will also continue to decrease costs and risk, preserving patient and hospital dollars for further research, in-depth analysis of existing conditions, and more extensive care options.
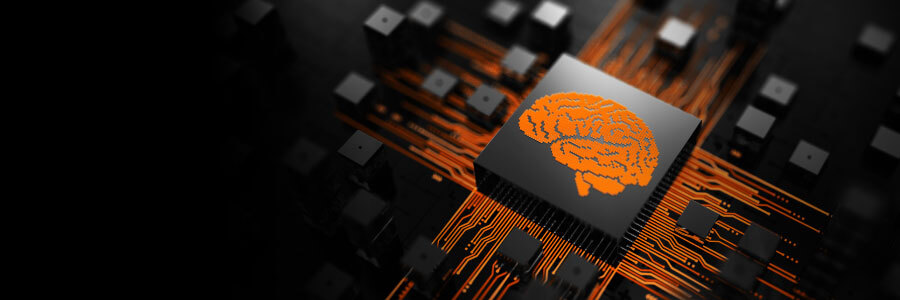